The device developer is tapping tech both old and new, explains Eko Health co-founder and CEO Connor Landgraf.
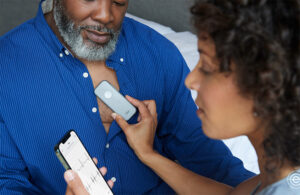
Eko Health’s algorithms use acoustic and ECG data collected by digital stethoscopes (pictured is Eko’s Duo stethoscope). [Photo courtesy of Eko Health]
Eko won FDA clearance for an algorithm powered by artificial intelligence — built with Mayo Clinic — using Eko’s digital stethoscopes for sound and electrocardiogram (ECG) sensing.
“The three legs of the stool for us are the device, the software, and then the disease-detection algorithms,” Eko Health co-founder and CEO Connor Landgraf said in an interview. “The devices have a longer manufacturing and development time given the hardware involved. We choose a set of sensors and capabilities, and then the goal is to be able to create as many meaningful disease-detection capabilities and algorithms using those sensing modalities. … One of the bigger focus areas for us has been how much signal and value can you extract from the combination of audio and ECG.”
Eko focused on acoustics and ECG to sense the heart’s rhythm and mechanical function — electrical activity of the heart muscle, then blood flow and the valve closure — and synchronize those two signals to provide hemodynamic parameters that wouldn’t be possible by just capturing each of those signals individually.
Eko’s digital stethoscopes — the Eko Duo and Eko Core 500 — have three ECG electrodes and synchronized acoustic sensing for simultaneous readings. Eko already had regulatory approval for identifying structural heart murmurs and arrhythmia detection.
The newest algorithm uses those same ECG and audio sensors to identify patients with reduced ejection fraction. Ejection fraction is typically measured from echocardiography, cardiac MRIs or other other imaging studies looking at the left ventricle’s size to estimate the percent of blood that moves out of the ventricle during contraction.
“In those cases where you have weak heart muscle, there are signals that are going to show up in ECG and there are signals that are going to show up in the acoustics that indicate not everything is well with the heart muscle and the left ventricle is maybe failing or insufficient,” Landgraf said. “We’re able to pick up those signals. … We believe it’s five or six different signals that the algorithm is able to identify and then come up with a likely value of whether the patient has normal or reduced [ejection fraction].”
The algorithm doesn’t produce a specific numeric value for ejection, but instead uses a cutoff threshold to say whether it’s above or below 40%.
“That’s the primary output from this algorithm,” he said. “It works quite well as an early detection tool for patients who might have a weak heart pump. And what we know about heart failure is these patients often have it for extended periods of time before they start showing real symptoms or the early symptoms are very subtle and many patients don’t really pay attention to them.”
When those patients aren’t diagnosed or treated, their disease progresses.
“We believe this technology can be a really powerful aid to the physician who’s evaluating a patient who has early, nonspecific symptoms,” Landgraf said. “We just want to get the right patients to cardiology sooner and make sure we don’t miss any patients. We’re not trying to diagnose them now. We’re trying to get them to the right diagnostics.”
Eko tapped tech new and old
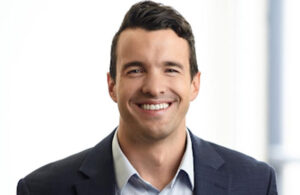
Eko Health co-founder and CEO Connor Landgraf [Photo courtesy of Eko Health]
“There’s a lot of signal here, but instead of being in the hands of the specialist, put it in the hands of the primary care physician or the frontline doc,” Landgraf said. “It’s really easy to do, and it can be quite accurate and aid them in that decision-making process.”
Eko tapped more recent tech advances to bring its hardware and software to market. Landgraf pointed to improvements in battery life that allow for power-hungry computing on a mobile device, growing acceptance of software and mobile technology in healthcare, and advances in wireless connectivity with high-quality Bluetooth.
Related: Tips on Bluetooth, batteries and Apple vs. Android from Eko Health
But the biggest enabling technology for Eko’s devices might be recent advances in machine learning, allowing the device developer to use very large data sets for pattern matching where there is a lot of variability in the signals
“With heart sounds, the patient may have a heart rate of 50 or 150 and the algorithms have to work well across those heart rate ranges,” he said. “There’s a lot of variability that the algorithms need to be able to take into account.”
Dataset size makes a “huge difference” for algorithm performance, Landgraf said. Eko’s now working with millions of data points and seeing payoffs as the dataset grows.
“We’re still seeing a linear correlation between dataset size and algorithm performance,” he said. “As the size — the number of recordings, the number of samples that we’re feeding the models — increases, we’re able to continue to drive improvements in the overall performance of the of the algorithm. That was not an unsurprising learning necessarily, but still kind of interesting to see that as you get into the millions of recordings in your training dataset it still continues to improve performance.”
Landgraf also emphasized the importance of labeling quality, ground truth quality and consistency in annotation.
“We like to think that medicine is extremely black and white and binary: disease/no disease, finding/no finding. The reality is there’s more variability in medicine than any of us would expect,” he said. “… Even in imaging, annotation in echocardiography is not consistent, especially when many of these measurements are hand-drawn. The estimation method used by the technician or by the sonographer during that cardiac imaging test can matter as well. If there’s a lot of human intervention — drawing boundary boxes, for example, on an echocardiogram image — anytime you add humans to that mix, you add variability and you have to account for it and try to quality control it as much as possible.”
ECG may be the most studied biosignal in human history, Landgraf said, and machine learning has advanced the field beyond a visual assessment of heart activity plotted on charts
“To analyze ECG, it’s always been what you could see with the eye and then the heuristics that you can build — AFib is P wave with irregularly regular heart rates and R peaks — and we had to develop the heuristic to describe an arrhythmia,” he said. “But with machine learning, we’re now finally moving past that point and saying, ‘What are the signals that a model can identify that may be invisible to the human eye?’ And when we crossed that threshold, you can do a lot of things you can’t do otherwise and you open up an entirely new set of possibilities.”
Eko eyes the lungs
When launching Eko, Landgraf said he and his founders wanted to build a Shazam for heart beats, referring to the smartphone app that can identify a song by sampling just a short audio clip.
“We’ve always said that Shazam for lung sounds also makes sense,” he said. “In addition to cardiac signals, the lungs and the the breath sounds in general have a huge amount of signal to indicate status about chronic respiratory conditions — asthma and pneumonia, chronic obstructive pulmonary disease (COPD) — that are particularly deadly and affect a very large number of patients. So I think chapter two for us is respiratory and pulmonary, and we believe that there’s a whole lot of signal that we can start to glean there.”
Eko is “actively working on a pretty large pipeline of those algorithms internally,” he said, though he declined to discuss timing.
“Then we think beyond that to vascular — blood flow in general. Identification of abnormal blood flow is really valuable in a lot of different places,” he continued. “And so we’ll probably continue to extend beyond that to other acoustic sound from the body.”
Related: How Medtronic’s using AI: Artificial intelligence insights and advice